Extracting product reviews from online shops has become one of the vital activities of e-commerce SEO and an essential source of competitive intelligence for any product marketer.
You will no doubt know that they are an essential part of the shopping experience for users.
But they also play an important role in Product Page Positioning (PDP) by adding genuine, free content about the product.
However, manually analyzing these reviews is a nightmare for anyone. Users, SEOs, e-commerce managers etc. can spend hours and days analyzing the content of these reviews.
Well, this is where our script comes in:
This Colab works as a product review extractor that, supported by AI, uses natural language processing (NLP) to extract valuable information from customer reviews and return them in a summary list of pros and cons.
What is a Product Review Extractor?
A product review extractor is a tool that uses Natural Language Processing (NLP) and Machine Learning (ML) algorithms to automatically extract product reviews from customer feedback on e-commerce websites.
These programs can analyze and classify reviews based on sentiment, keywords and topics, among other things.
As programmed.
In our case, the script we have prepared allows us to automatically generate a list of pros and cons, which users have reviewed in order to use it as a table in our product descriptions.
Or also to identify problems in the offer or service to improve products and services.
In fact, this tool can be customized to meet the specific needs of each professional profile, company or user interest.
The importance of evaluating product reviews in SEO
Analyzing product reviews is essential from an SEO point of view. There are several reasons for this:
1. Improve search engine positioning:
Product reviews can play an important role in improving your search engine rankings.
This is because search engines like Google use customer reviews as a signal of the quality and relevance of your product pages.
By analyzing these reviews and incorporating relevant keywords and phrases into your product descriptions, you can optimize your content for search engines and improve your ranking.
2. Provides valuable user-generated content:
User-generated content, such as product reviews, is invaluable because it adds credibility to product listings, helping to establish trust with potential customers.
From an SEO point of view, this is invaluable.
By analyzing these reviews and incorporating customer-rated attributes into product descriptions, it helps to highlight and improve conversions.
Improving the user experience also has an impact on search engine rankings and driving traffic to your website.
3. Helps to identify and address customer concerns:
Finally, analyzing product reviews also helps to identify and address any customer issues with products.
By understanding what they like and dislike about them, we can make improvements and updates to them.
Or detect problems related to delivery service, or product quality.
Overall, analyzing product reviews is essential not only for SEO, but for any online shop manager.
By understanding what customers are saying, you can optimize your content and provide the most valuable information, improving your response to users’ search intentions and clearing objections to improve search engine rankings and conversions.
What you need to extract product reviews from a URL
Actually, all you will need are 3 things:
- Your OpenAI API Key
- The product URL to extract the reviews
- And install the Google Colab dependencies.
At this point, we have prepared an explanatory video created by our colleague Luis, who has also prepared the script to make everything much easier for you.
If you have never used a Colab before, we recommend you to watch it.
You can watch it right here:
This content is generated from the audio voiceover so it may contain errors.
Hello everyone! Welcome to a new video from iSocialWeb. I’m Luis Fernández, and we continue with our series of videos on Artificial Intelligence applied to SEO and websites. In this video, which I believe will be very useful for both agency webmasters and the regular viewers of this channel, as well as for affiliate marketers who may have their own affiliate niches or AdSense sites, we’re going to explore a rather simple script. My aim is to give you an idea that this is doable, it’s easy to accomplish, and you can adapt it to your projects. As always, it’s quite straightforward to program everything, and if you’re not familiar with coding, you can always rely on Chat GPT to assist you in adapting the code to your specific use cases.
What we’re going to do in this case is to scrape reviews from the competition or from a website that isn’t our own. If it’s our own website, we simply can’t download and use the CSV, uploading it directly, and saving ourselves the scraping part. We’re going to use GPT in this case, version 3.5, because it performs perfectly and is much more cost-effective and faster. However, you could use GPT-4 or a future version coming out soon because it’s progressing rapidly. We’ll ask it to summarize the reviews for us. With this, we’ll obtain a list of pros and cons based on real user reviews. This is perfect for creating affiliate articles with product reviews, as well as for expanding product descriptions in our eCommerce store based on user reviews. Often, these reviews are paginated, hidden, or not displayed directly.
It’s also very useful for populating eCommerce stores where we want to add a product that our competitors have, and we need a product description. In this case, we can review our competitors, extract their reviews, and create a description based on real opinions. All of these scenarios can be applied in various ways; there’s always room for customization. I’ll show you the code, and then it’s in your hands to create some really cool stuff. As always, we work with Collage, and the link will be in the video description. I won’t go into detail about the code, but essentially, we’re going to use two libraries: “tiktoken” for counting tokens and setting limits, and “OpenAI.”
Here, we import everything. You need to input your data here; you put your API key here, select the model here, and set the maximum number of tokens. Remember that GPT-3.5, for example, has around 4,048 maximum tokens, if I recall correctly, and you need to leave some room for the response and system input, as mentioned earlier. Next, you enter the URL directly. I chose an eCommerce website that’s fantastic and selected products from guitar sales. As you can see, we scroll down, and we have reviews on Zoman. There are some really detailed reviews; musicians really put a lot of effort into the content. Here, you can choose Zoman for this case. The scraping is set up for Zoman, but you can easily adapt it to Amazon or any other eCommerce platform if you wish, like PCCOMPONENTES or others.
If you’re using your own store, you can skip all this scraping part. It’s marked as scraping here; you can simply upload a CSV or the reviews directly and work with your product’s constraints. Once you have the URL, we’ll set some headers. If you want to do this more professionally, I recommend using proxies and a header generator, or even a library like “Apio” to manage all of this. Another important thing is that if you’re scraping Amazon, it can quickly block you, especially in this case where we’re using BeautifulSoup, which is not the most complex crawler and doesn’t mimic any search engine. If you use Selenium with a couple of proxies, you can scrape whatever you want. Here, we have a couple of utility functions: getting the HTML of the product, the reviews. We get the HTML of the page, which you can see here. It’s just code lines. Keep in mind that this is a crawler and doesn’t generate any kind of browser. If you want to scrape pages with JavaScript, you’ll need a crawler that can render JavaScript. Once you have the rendered or downloaded HTML, you simply scrape it, extract the product and the reviews. As you can see, getting the product name is as simple as grabbing the h1. This will work on most well-made websites because they typically have an h1. If there are multiple h1 tags, we’ll take the first one. As for the reviews, they depend on the specific webpage, and they can vary.
You can use Chat GPT to inquire, especially if you’re not well-versed in programming. Here, you can see all the reviews we’re targeting. I can press F12 or inspect, and I can look through here to find the reviews. We’re capturing this, so there’s no need to dig into the code. We can see that the reviews will come out like this. “Review test” is the text we’re capturing. So, if you notice in the code, we’re looking for a div with the class “reviewtest.” In your example webpage, instead of “review test,” it might be “review” or something similar. You can adapt it. There are different scenarios, but I believe that with a bit of dedication and Chat GPT, you can do this easily. After running all of this, I’ve left some print statements here to clarify what you’re doing. You can see the reviews like these. We’ve extracted four reviews, and this is the product name. Next, we make the typical call. In this case, we’re going to count the number of tokens. These are slightly complex functions, taken from the “tiktoken” documentation. You don’t need to understand them, just know that they return the number of tokens for a string and for messages. What we’re doing is limiting them. I set the default limit to 1,000, but we’re going to use the 2,000 tokens we marked above. We’re limiting it here and generating the prompt. We format the reviews, and this generates a prompt like this: “Summarize the following list of reviews for [product name], indicating the pros and cons based on the following reviews:” Colon. And here’s the list of reviews.
I left this here because often, when scraping a large website like Amazon, you’ll have reviews in multiple languages. You can manually filter them in the code or simply add this and trust that 90% of the time, it will do it correctly and leave it in Spanish. Then we make the call. It’s quite simple. It’s just these five lines of code. We input the model, which we selected above, in this case, GPT-3.5 Turbo. This is the system message, which you can customize to your liking. And a prompt, which I recommend playing around with a bit. I’ve left you with the most basic one here, but you can personalize it by product type, store type, etc. Then we capture the content and the response. This is the response it provides. You can see that for this “Hard Livento in HB 10g,” it says that the price is very affordable, it’s robust and durable, small and lightweight, easy to handle. You can see that it even mentions good service for beginners in the world of guitar. These responses are very real pros and cons. You could generate a list of questions and answers or request recommendations for a buying guide. Once you have all the groundwork, just know that you have to create with those reviews, change the token, not the prompt, and you can do whatever you want. And that’s it. I hope you like it. It’s a pretty powerful tool, and it works very well. If you have any questions, you know, the whole playlist is available on the iSocialWeb YouTube channel, and we’re in the comments to assist you. Greetings, and thank you very much.
As you can see, it’s all very simple.
However, we recommend you not to use it in an online shop like Amazon, eBay, or harpersbazaar because they usually have anti-scraping systems and probably the libraries we have used in this Colab are not enough to avoid such systems.
For these cases we recommend you to use Selenium and a couple of Proxies, you can scrape as much as you want without being blocked.
In any case, use it wisely and without abusing it to avoid crashes.
How our automatic review extractor works
Our e-commerce product review extractor is based on natural language processing with OpenAI’s GPT.
We have included the tiktoken libraries to count tokens and avoid exceeding the maximum limit of 4048 tokens in GPT3.5.
Remember that you need to leave room for the response, so don’t reach the limit and don’t forget to insert your API KEY and the URL to parse.
It is also possible to change the model and the number of reviews to analyze.
In the video example, we have used 4, but more can be analyzed.
Here you can see an image of the generated prompt:
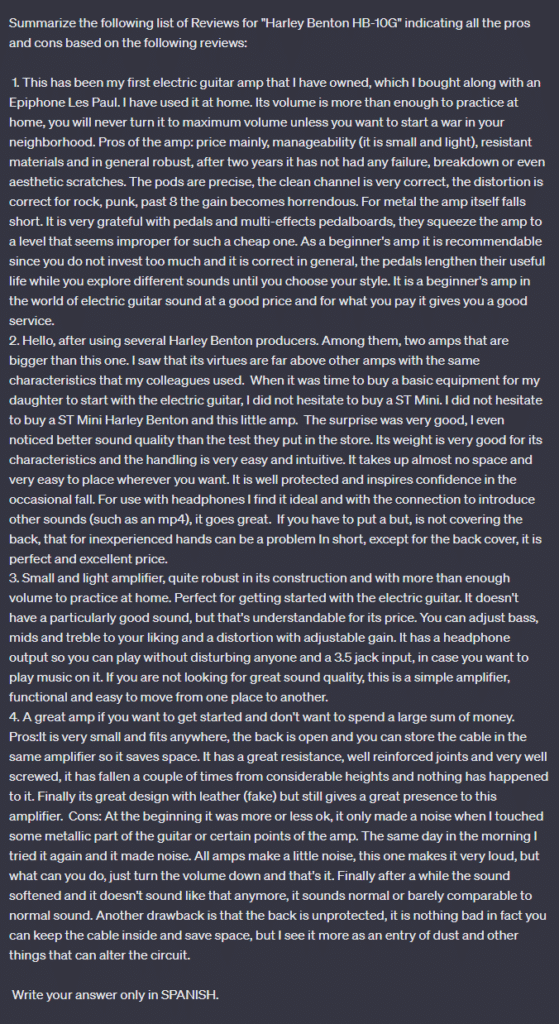
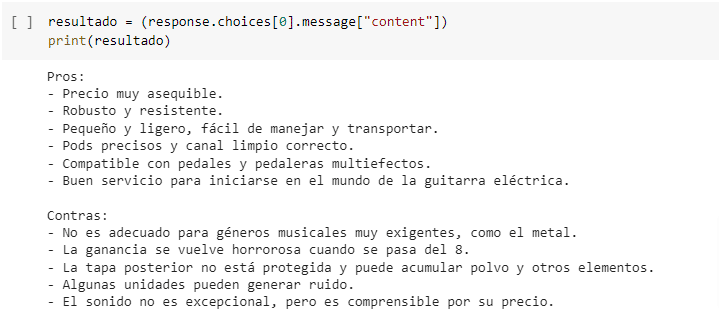
As you can see, at the end, you will get a list of pros and cons for the page provided in Spanish.
Although you can configure the Colab or use a text translator into English.
The truth is that with our script it is very practical and simple.
You only need to select the URL from which you want to extract the data, enter it in the Google Colab together with your OpenAI API Key and press the button to process the information.
This way, you can quickly analyze any URL and obtain a summary with all the strengths and weaknesses of the product to:
- Draw conclusions.
- Understand what users value
- Identify potential improvements of the product/service
- Create a pros and cons table for your product description.
- Incorporate valuable attributes that you have not considered in the content.
Benefits of using a product review extractor
Increased accuracy and efficiency in extracting product reviews
AI product review extractors can extract product reviews from large amounts of customer feedback accurately and efficiently, saving companies time and money.
They are also able to recognize patterns and trends in customer reviews, allowing companies to identify common problems and themes.
Ability to analyze large amounts of data quickly
Analyzing customer feedback, providing companies with up-to-date information on product performance, is another capability of these applications.
The information can be used to improve product development and customer service.
Cost-effective compared to manual extraction methods
AI product review extractors are significantly more cost-effective than manual extraction methods, reducing the need for human resources and increasing productivity.
Improving product page content and customer experience on e-commerce websites
An extractor can provide companies with information about the likes and dislikes of their customers, allowing them to make informed decisions about product development and marketing.
This information can also be used to optimize product pages and provide customers with a better shopping experience.
Better understanding of the likes and dislikes of your supply side
They help organizations identify areas for improvement in their products and services, enabling them to make changes that improve customer satisfaction and increase sales.
Other use cases and applications
There are several use cases for an e-commerce product review extractor with AI apart from the ones mentioned above.
Some of them have a lot to do with:
- Market research: analyze customer opinions and feedback and gain valuable information on market trends and customer preferences.
- Product improvement: Use the information generated by the extractor to identify product problems and improve product quality, durability, performance and other aspects of the product.
- Competitor assessment: Companies can use an AI-enabled e-commerce product review extractor to analyze customer reviews of competitors’ products and compare them to their own products.
- Business decision-making: use the information generated to make informed decisions about product improvement, customer satisfaction and marketing strategy.
Potential challenges and limitations of AI product review extractors
While AI product review extractors offer significant advantages, there are also potential challenges and limitations to consider, including the following:
1.Ethical concerns regarding the use of AI technology
There are ethical concerns regarding the use of AI technology, especially with regard to privacy and bias. It is essential to ensure that the tool is used in an ethical and transparent manner.
2.Limitations in accuracy and efficiency
AI product review extractors may not be entirely accurate or effective, especially when analyzing complex customer reviews. It is essential to ensure that the tool is continually updated and maintained to ensure its accuracy and effectiveness.
3.Need for continuous updates and maintenance
The Extractor requires continuous upgrades and maintenance to ensure that it remains effective and up to date with the latest technologies and customer needs.
Bottom line
In general, the use of a product review extractor provides valuable product information in a short time.
They make possible to perform tasks that were previously only available to a few companies and organizations with large marketing budgets.
Now, however, the playing field is more level.
Think about it for a moment:
Before, to get a list like the one provided by our extractor, you would have to:
- Search for review websites or e-commerce websites with user reviews
- Read the reviews available on e-commerce websites. You could use filters or sorting options to display only the most relevant reviews based on criteria such as date, rating or relevance.
- Identify common topics or issues using tools such as word clouds, text analysis software or manual tagging to categorize reviews.
- Extract pros and cons mentioned in the reviews based on the identified topics by deploying a spreadsheet or similar tool to group the pros and cons for future analysis.
- Analyze the data looking for patterns or trends using statistical analysis software or visualization tools to create charts and graphs.
- Draw conclusions based on the data analyzed.
As you can understand, this process is a drain on time and resources that you can invest on other tasks.
Thus, we hope you see how useful our extractor can be.
Now, we are sure you do!
Would you like us to help you optimize your product descriptions with AI?
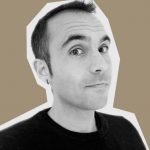
Co-CEO and Head of SEO at iSocialWeb, an agency specialized in SEO, SEM, and CRO that manages over +350M organic visits per year with a 100% decentralized infrastructure. Also, the founder of Virality Media, a company with over 150 million monthly active visits across various sectors and industries. A Systems Engineer by training and an SEO by vocation. An avid learner, AI enthusiast, and dreamer of prompts.
- Este autor no ha escrito más artículos.